1. Headline facts about 5G
- Maximum 5G download speeds of greater than 750Mbps
- Average 5G download speeds of roughly 90Mbps-230Mbps
- 17 mobile operators have so far launched 5G
- At least 114 major towns and cities have 5G with varying coverage
- 5G will support around 3 million connected devices per square mile
- To get 5G you need a 5G phone, 5G plan and 5G signal
2. What is 5G?
5G (which stands for fifth generation) is the latest step in mobile technology, following on from 4G before it and 3G before that, and like the jump from 3G to 4G, you’ll be getting far higher speeds than on any of the technologies that came before.
We’re talking both upload and download speeds, and we’ve got a whole guide to 5G speeds and what they allow you to do, but speed isn’t all it has going for it. This latest generation of connectivity also offers lower latency (the time a network takes to respond to a request), promises greater capacity for users, and will enable and improve all sorts of related tech, such as the Internet of Things (IoT).
3. How does 5G work?
5G uses mobile masts and other infrastructure (such as small cells) to transmit a signal to your smartphone (or other 5G device). So if you’re in range of a 5G mast, then your phone will be able to get a 5G signal.
This process is much the same as the one used for 4G and 3G, so there’s no real change to how you get 5G on your phone. Indeed, 5G simply means ‘fifth generation’ – so it’s the fifth generation of the system we’ve been using on mobile phones since day one.
However, behind the scenes there are some differences. 5G (like 4G and 3G) is carried on various frequencies of spectrum, but the frequencies used by 5G tend to be higher, as high frequencies are available in greater capacity, which is necessary for delivering a reliable 5G network.
These frequencies are also shorter range than the ones used for 4G and 3G though, which means more masts and other infrastructure is required to blanket the UK in 5G.
As well as different spectrum and additional infrastructure, new technologies are also involved in 5G networks. These are numerous, but a key one is Massive MIMO (multiple-input multiple-output), which allows the use of multiple antennas to send and receive multiple signals at the same time. By sending more data at once and through multiple antennas, speed and stability can be increased.
You can click through to the guides linked in this section to learn more about key 5G technologies, but as a user the experience will be much the same as on 4G – just a whole lot faster.
4. What are the benefits of 5G?
4.1 Much faster speeds
5G is of course much faster than 4G. Where 4G download speeds average around 36.4Mbps (based on data from Opensignal), on 5G you can currently expect average speeds of between around 90-230Mbps – depending on network and location, with peak speeds of over 1Gbps (where they generally top out at around 100Mbps on 4G), and speeds will improve ever more as networks mature.
Network type
|
Average / max download speeds
|
Time to download a full HD film
(based on average speeds)
|
3G
|
8/20Mbps
|
Over a day
|
4G
|
36.4/100Mbps
|
Over 7 minutes
|
4G+
|
42/300Mbps
|
2.5 minutes
|
5G
|
90-230/10Gbps (theoretical)
|
4-40 seconds
|
Already we’re seeing top 5G download speeds of over 750Mbps, but theoretically we could see speeds of up to around 10Gbps on 5G eventually.
4.2 Lower latency / response times
Speed is the single most talked about advantage of 5G, but it’s not the only one, with low latency being another big factor, and one which ties into speed. Latency is how long a network takes to respond to a request, so if it’s high then it can take a while for things to happen even with good download speeds.
On 4G, latency averages around 35-50ms (milliseconds), while on 5G, the average is around half that currently and will go as low as 1ms ultimately.
Those actual 5G latency figures in the chart below are taken from 2021 Ookla data. This report found that EE and Vodafone each had a 5G latency of 29ms, while O2’s was 31ms and Three’s was highest - and therefore worst - at 33ms. Beyond that, however, RootMetrics looked at 5G latency in central London in late 2020 and found that Three’s was just 17ms, Vodafone’s was 34ms, and EE's was 45ms. O2 meanwhile didn’t have 5G in London when these tests took place.
These two reports have interestingly conflicting results, as Three’s latency is best in one and worst in the other, but as the latter only factors in central London we can assume that Three has excellent 5G latency there but less impressive 5G latency elsewhere.
The 3G and 4G latency figures in the chart below come from various sources, but as an example, RootMetrics data from April 2020 found that the lowest average 4G latency of any network was 36ms, while on 3G it was 58.2ms.
In any case, you can probably imagine many of the differences these speed and latency improvements can make in daily use, but further down you’ll find some key examples, including both obvious applications and those you might not have considered.
Network type
|
Approx. latency (ms)
|
3G Network
|
~55-65ms (actual)
|
4G Network
|
~35-50ms (actual)
|
5G Network
|
29-33ms (actual) /1ms (theoretical)
|
4.3 Greater capacity for users
5G spectrum is available in greater capacities than 4G spectrum, which in turn means there’s more capacity for the end user, so a larger number of devices can be connected at high speeds. That in turn should make it more reliable than 4G.
To put this into perspective, with 4G there can be around 2,000 connected devices per 0.38 square miles, whereas 5G can theoretically support up to 1 million connected devices per .38 square miles.
The spectrum frequencies in use by both technologies can be seen below – note that not all networks use all of these frequencies, and that in general the higher frequencies have greater capacity available. Note also that currently only the 3.4GHz and 3.6-4GHz bands are in use for 5G – the other listed frequencies are likely to be made available in future auctions though.
4G frequency spectrum
|
5G frequency spectrum
|
800MHz
|
700MHz
|
1.4GHz
|
3.4GHz
|
1.8GHz
|
3.6-4GHz
|
2.1GHz
|
8GHz
|
2.3GHz
|
24GHz and higher
|
2.6GHz
|
|
4.4 Improved reliability
As noted above, the greater capacity available will also help make it more reliable than older network technologies, meaning dropped calls should be a thing of the past, your network experience should be good even in busy places, and with that increased reliability, it will be suited to things where reliability is essential – such as self-driving cars and remote surgery.
4.5 More flexible
Some of the new technology in 5G allows for network slicing, which means a single physical network can be divided into numerous virtual networks suited to different needs, rather than having to rely on a one-size-fits-all network, which won’t be the ideal fit for many situations.
5. Is 5G available in the UK?
5.1 Which operators have launched 5G so far?
At the time of writing, the UK’s four main networks (EE, Three, O2 and Vodafone) have all launched a 5G service. A large number of MVNOs (mobile virtual network operators) have too, namely BT Mobile, Tesco Mobile, Sky Mobile, Giffgaff, Virgin Mobile, Asda Mobile, iD Mobile, Lebara Mobile, CMLink, Lyca Mobile, Smarty Mobile, Talkmobile and VOXI – though they each rely on the coverage of one of the main networks.
Coverage on the main networks varies and is increasing rapidly, but most of the UK’s major cities now have at least partial 5G coverage on at least one of them, and many have coverage from all.
For example, Birmingham, Bristol, Cardiff, Glasgow, Leeds, Liverpool, London and Manchester all have a 5G footprint from all four main UK networks, as do a vast number of other places.
5.2 Check 5G coverage in your area
5G isn’t yet available everywhere, and even within towns and cities that do have it, coverage isn’t yet comprehensive and may in some cases be sparse, so it’s worth doing a proper check of coverage in your area. You’ll find our 5G coverage checker below, which lets you enter your postcode to find each network’s coverage in your area, and also contains in-depth details of each network’s 5G coverage across the UK.
5G Coverage Checker
Find out which networks have launched 5G in your area,
or when it is coming to your area.
5.3 When will the rest of the UK get 5G?
The UK’s networks are rapidly expanding 5G coverage across the UK, but at the time of writing (April 2023) it will probably still be a while before this coverage rivals 4G.
Exactly when specific places will get it isn’t known for the most part, but as a general rule, the bigger and more urban a location the sooner it is likely to get it. You can check out our 5G coverage pages for the latest details on which locations have 5G already.
6. How do I get 5G?
6.1 What do I need?
To get 5G you need three things: a compatible device, plan, and 5G coverage. Click the link below for an in-depth guide to getting it. Beyond that, you’ll find information on coverage above (and on our coverage pages) and information on phones and plans below. Read our full guide: 5G upgrades - how to get 5G?
6.2 What 5G phones are available?
There’s a huge and growing number of 5G phones available. These include the iPhone 14, iPhone 14 Plus, iPhone 14 Pro, iPhone 14 Pro Max, iPhone SE (2022), Samsung Galaxy S23, Samsung Galaxy S23 Plus, Samsung Galaxy S23 Ultra, the Samsung Galaxy S22 range, the Google Pixel 7, the Pixel 7 Pro, the OnePlus 11, and many others, including a lot of mid-range and affordable phones.
6.3 Is there an iPhone 5G?
Yes, the entire iPhone 14, 13 and 12 ranges support 5G, as does the iPhone SE (2022). That means you can get 5G with an iPhone 14, iPhone 14 Plus, iPhone 14 Pro, iPhone 14 Pro Max, iPhone SE (2022), iPhone 13, iPhone 13 Pro, iPhone 13 Pro Max, iPhone 13 Mini, iPhone 12, iPhone 12 Pro, iPhone 12 Pro Max, and iPhone 12 Mini. No other iPhones currently support 5G, but we’d expect that going forward it will be a standard feature for Apple’s handsets. Read our full guide: Which iPhones support 5G?
6.4 Can I get a 5G SIM Only deal?
Absolutely. At the time of writing, EE, Vodafone, Three, O2, Asda Mobile, iD Mobile, Lebara, Lyca Mobile, Smarty, Talkmobile, Sky Mobile, Giffgaff, Virgin Mobile, Tesco Mobile and VOXI all offer 5G SIM Only plans, and more networks are likely to start doing so over time.
6.5 Is 5G broadband available?
Yes, you can get both 5G home broadband and mobile broadband – the difference being that mobile broadband devices tend to be battery-powered and portable, whereas home broadband ones are designed to be plugged in and provide long-term broadband to your home.
One or both of these services is available on a number of networks, including EE 5G home broadband, Three 5G home broadband, and National Broadband, and they’re worth investigating, as they offer speeds that can rival fibre, coupled with a faster, simpler setup and potentially lower prices.
7. What can 5G do?
5G will change our lives in a multitude of ways. We’re already seeing the early benefits of it, but there’s much more to come. First up, there’s some obvious benefits: with all that speed you can download content a lot faster, which in turn makes 4K, 8K and even higher resolution video far more viable, as when streaming it there’ll be minimal buffering, and when downloading you won’t have to wait an eternity.
Below we’ve listed some other big benefits of 5G.
7.1 5G gaming
5G could allow for far more graphically rich games on mobile, ones that truly approach console quality, as while the file sizes will be huge, it will be able to cope.
Of course, smartphone hardware might not be able to keep up with the demands of console-quality games, but it might not need to, as with 5G, game streaming is also becoming far more viable, meaning your able to stream the entire game over the internet, like you’re already used to doing with music and video.
This not only means you won’t need to download your games, but more importantly means all the processing can be done in the cloud, so you won’t need a high-end handset for otherwise demanding games. We’re already starting to see this with services such as Xbox Cloud Gaming.
The speed and especially the low latency of 5G will also transform online gaming, as no longer will there be any lag when playing on mobile.
7.2 Virtual reality, augmented reality, and holograms
As well as higher resolutions, 5G will allow for better, more immersive virtual reality (VR) and augmented reality (AR) experiences, whether in gaming, live events, or a number of other things.
Imagine being able to be virtually present at a football match or the front row of a live concert, wherever you are, simply by donning a VR headset. This will become possible even when you’re not tethered to a fibre broadband connection, and the experience should remain smooth and immersive.
We will likely also see augmented reality displays projected over a car’s windscreen, highlighting businesses and potential hazards on the road ahead, or projecting direction arrows from your car’s sat-nav system. 4G isn’t fast or reliable enough to make this work effectively in most places, but 5G will be.
It’s also expected to usher in the era of holograms. Vodafone for example has already carried out a holographic call over 5G, with a live hologram of a footballer being projected across the UK, so they could have a conversation with a fan almost as if they were there in person.
The speed and latency of 5G can handle all the data required for that, and while we’re probably years off the required hardware being commonplace, this is one way it could transform your life in the future – making it seem like you’re in the same room as the person you’re talking to, even if they’re really at the other side of the world.
7.3 5G home broadband
Home broadband still isn’t in the state it should be, with many people still unable to get super-fast connections, and even our fastest fibre speeds trailing some parts of the world, but 5G will provide a reliable, accessible alternative.
Since it has the potential to be every bit as fast as fibre broadband, places that have 5G coverage but only have slow broadband could benefit enormously by switching to 5G for their home internet. Some networks are already offering 5G home broadband in select locations, so it’s clear that this will be a major, transformative use of the tech.
The nature of this next-gen connectivity also means that 5G home broadband should be cheaper to install and maintain, and easier for users to set up in their homes, making it potentially cheaper and simpler than fibre broadband too. Check out our 5G vs fibre guide for more about this.
7.4 Remote working
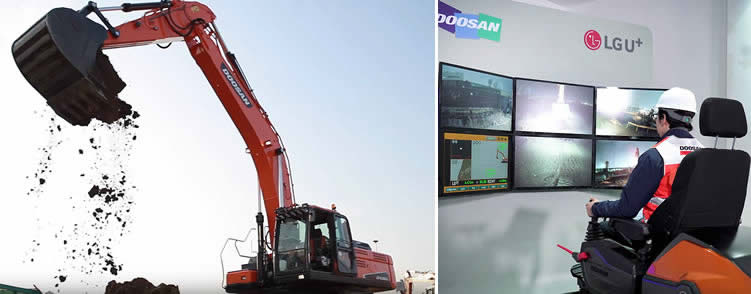
Given that 5G could rival the speed of fibre broadband, it’s of course also ideal for remote working. Enabling you to work as if you were at home or the office wherever you are, even going so far as to let you take lag-free 8K video conference calls.
But that’s just the beginning. Construction workers will be able to operate excavators and cranes from thousands of miles away, doctors will be able to consult with patients and paramedics from afar, and surgeons could even control surgical robots remotely.
In these ways it will bring us all closer, and all but eliminate the work-day commute.
7.5 The Internet of Things
The Internet of Things (IoT) isn’t a new concept, but it has been rapidly growing over the last few years and with 5G becoming available it’s set to really take off.
The IoT encompasses all the ‘smart’ and connected devices we’re starting to see in homes and beyond, from smart light bulbs and thermostats, to connected kettles, security cameras and more, most of which can be controlled by an app on your phone, or by your voice when paired with an AI Assistant like Amazon Alexa.
In some cases they can even be automated, with lights for example turning on when you enter a room and turning off when you leave it. They do this in part by communicating with each other. For example, motion sensors at a room entrance could tell the lights when you’ve entered.
But it’s not just homes – we’re also starting to see the IoT connect cities, cars, health care, factories and more, to make life easier, smarter and more automated.
5G comes into this because its high speed and reliability along with its low latency is necessary to keep growing the IoT. Before you know it, almost every device that could possibly be connected will be.
7.6 Smart cities
Smart cities will in many ways be an extension of the IoT, taking these devices beyond homes and out into the world to make cities, well, smarter.
Think traffic lights that can adapt automatically based on traffic flow, automatic deployment of road repair vehicles when potholes are detected, smart power distribution, so buildings aren’t being lit when they’re empty, and rubbish bins that know when they need emptying and alert the relevant people, all leading to cleaner, safer, more efficient cities, and all with minimal human oversight.
This, even more than IoT devices in the home (which can often rely on fast fibre broadband), really needs the speed and coverage of the latest mobile connectivity to work reliably.
7.7 Cars of the future
.jpg)
According to a report from Gartner (a research company), 94% of cars will be connected to 5G by 2028. With that they’ll then be able to communicate and share data with other vehicles and connected infrastructure, avoiding accidents and traffic as a result, and telling smart cities where traffic is building up, so routes for other vehicles can be adjusted.
That’s just a few ways a 5G connection will help cars of the future. They could also use it to power air quality sensors, in-car entertainment systems, AR dashboards, and even full vehicle automation.
That’s right, it could also play a large role in self-driving cars, with its speed and reliability key to them rapidly sending and receiving information about the road and other vehicles.
7.8 Drones
Drones have become quite popular in recent years, but 5G could massively increase their capabilities and safety.
One example of this is drone racing, with 5G allowing you to put on a virtual reality headset and get a drones-eye-view of the race, making it far more immersive than just controlling the drone from a little screen.
This, as with other VR, is a lot more viable over 5G due to the high speed, low latency and general reliability of the tech. With an inferior connection there could be a delay in your inputs and the view of the action, potentially causing you to lose the race or even crash the drone.
It could also be the key to making drones safe enough to be operated beyond the pilot’s line of sight, as it is a speedy and stable enough tech to track all aircraft in an area, so there’s no danger of a collision.
8. FAQs
What does 5G really mean?
5G simply means ‘fifth generation’, because it’s the fifth generation of mobile networks. So it’s a generational leap from 4G, which itself was a generational leap from 3G.
But the move to 5G could prove even bigger than the move to 4G did, as it goes beyond higher speeds, allowing us to connect and automate ever more things, and potentially underpinning Industry 4.0 – the fourth industrial revolution.
For more on this, check out our guides to the Internet of Things and how 5G will reshape business.
What is the difference between 4G and 5G?
From a user perspective, the main difference is that the latter typically offers much higher speeds and lower latency. That’s what you’ll notice when using it. Thanks to these qualities, it will also help grow the Internet of Things – leading to ever more connected and smart devices.
Behind the scenes, it uses different frequency bands to 4G, and different technologies, such as Massive MIMO and small cell infrastructure. But as an end user the experience of connecting to a 5G network will be much the same as a 4G one, you’ll just benefit from lightning fast speeds once you’re on it.
Can I upgrade to 5G?
Absolutely – though if you’re currently tied into a contract you may need to wait for this to end.
Then it’s just a case of switching to a 5G phone and plan. Assuming you’re on a network which currently has 5G coverage you should be able to get both of these things without jumping through many hoops – you can just upgrade your plan as usual, opting for a 5G one this time around.
You can find full details of upgrading in our 5G upgrades guide.
Will I need a new phone to get 5G?
If you’ve upgraded your phone relatively recently and opted for a 5G handset (which most flagships from 2020 and beyond, and most mid-range phones from 2021 and beyond are) then you’ll be good to go, otherwise you will need a new phone.
The good news is that there’s a large and growing number of 5G phones, such as the Samsung Galaxy S23 and iPhone 14, with many flagships now supporting the tech, and even a growing number of lower end phones, such as the OnePlus Nord 2, but most older handsets don’t support it.
Over the coming years we’d expect 5G to start being supported as standard on new phones – even the very cheapest ones, but you’ll still need to upgrade if you currently have a 4G phone.
Does 5G use more data and how much?
No, it doesn’t use any more data than 4G or even 3G. So for example if you’re streaming a 1080p video it will take the same amount of data to do this over 5G as over 4G.
That said, because data moves so much faster over 5G, it’s a lot more viable to download big files and stream higher quality content, so a lot of people may find themselves changing their usage habits in ways that do consume more data.
Indeed, in a September 2020 Opensignal study, the company found that UK users on average used 2.5x more data on 5G than 4G. So you probably will use more data too, but you don’t have to.
For more information read our comprehensive guide: Does 5G use more data?
How will 5G impact businesses?
Potential benefits of 5G for businesses include new products and services and even whole new industries that just weren’t possible with 4G technology.
It will also aid in things like remote working, through things like 8K video conferences and holographic calls, along with allowing for smart and automated factories, driverless trucks, virtual reality tourism, improved engagement with products through augmented reality, remote and robotic surgery, and more.
Network slicing meanwhile could allow for networks that are tailored to the needs of individual businesses, and it could aid rural businesses, which are currently often stifled by poor network connections and slow broadband.
And these are just a few examples of its role in business.
For more on the benefits of 5G for businesses, check out our ‘How 5G will reshape business’ guide.
Does 5G pose health risks?
The short answer is no. There have been extensive studies into the effects of mobile phone usage and the radio frequency (RF) fields that they give off, and no convincing evidence has been found that they affect health.
Most of these reports are for 4G and older tech rather than 5G, but the latter is a similar concept, just higher frequency, and talking about 5G specifically, the Department of Health and Social Care (reported by Ofcom) has said:
“Exposure to radio waves has been carefully researched and reviewed. The overall weight of evidence does not suggest devices producing exposures within current guidelines pose a risk to public health.”
Ofcom specifically has found that electromagnetic field (EMF) emissions from 5G are at most around 1.5% of the maximum safe level, while the ICNIRP (International Commission on Non‐Ionizing Radiation Protection) has spent seven years coming up with new mobile guidelines which will be followed to ensure all forms of 5G are completely safe.
The UK government meanwhile has added that:
"It is possible that there may be a small increase in overall exposure to radio waves when 5G is added to an existing network or in a new area. However, the overall exposure is expected to remain low relative to guidelines and, as such, there should be no consequences for public health."
For more in depth details about the safety, check out our ’How safe is 5G?’ guide.
Is Huawei involved in UK 5G networks?
UK networks have been banned from buying Huawei equipment as of the end of 2020, and by the end of 2027 its 5G equipment must be completely removed from UK networks, until then though some networks will be using some of Huawei’s tech.
Huawei phones are also still being sold in the UK, as these aren’t seen as a security risk in the way that its infrastructure is.
This move to ban Huawei gear though does mean that the UK’s rollout of the speedy new tech could be slowed – which could cost the UK billions.
Will 5G be available in rural areas?
Yes. As with 4G you should ultimately be able to get 5G in most parts of the UK, whether urban or rural. However, again as with 4G it’s likely urban areas that will get widespread coverage first. Indeed, most of the places that currently have 5G are towns and cities.
This is to be expected, since more people can be given coverage in a smaller space, making roll outs in these areas a more efficient use of a network’s resources initially, but rural locations are sure to follow.
If you’re not sure whether you have 5G where you are now, make sure to check 5G coverage.
Did 5G cause Covid-19?
No. The claims that it did are nothing more than conspiracy theories, with no basis in science. As noted above, 5G is safe, and it also doesn’t work in such a way that causing something like Covid-19 would even be theoretically possible.
Numerous experts and organisations have dismissed the links, with the UK’s four main mobile networks saying in a collective statement for example that “there is no scientific evidence of any link between 5G and coronavirus. Fact.” The Word Health Organisation meanwhile states that "5G mobile networks DO NOT spread COVID-19" as "viruses cannot travel on radio waves/mobile networks".